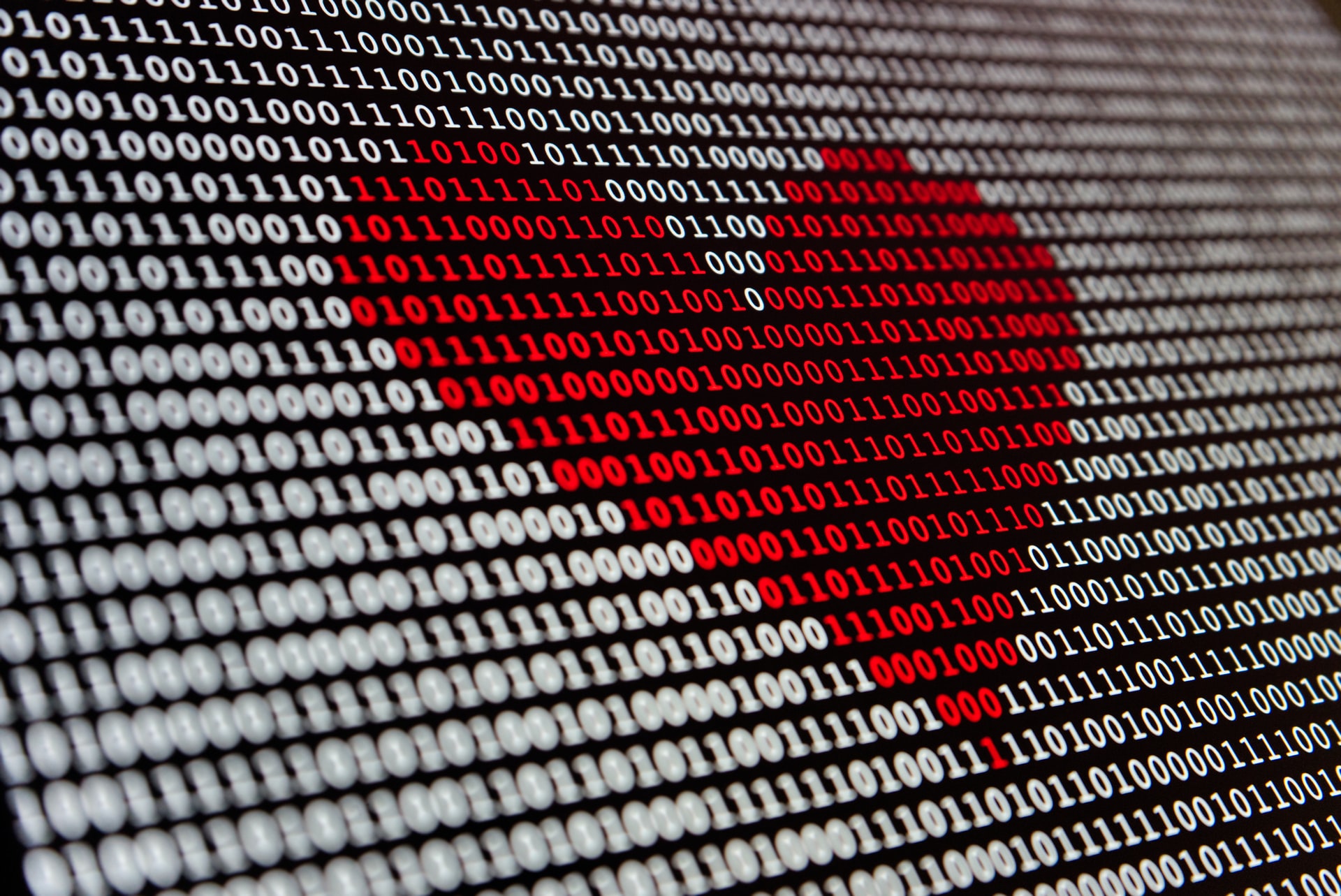
Researchers from OpenAI, Google Brain, Intel, and 28 other leading organisations have published a paper which proposes a ‘toolbox’ for verifying AI ethics claims.
With concerns around AI spanning from dangerous indifference to innovation-halting scaremongering; it’s clear there’s a need for a system to achieve a healthy balance.
“AI systems have been developed in ways that are inconsistent with the stated values of those developing them,” the researchers wrote. “This has led to a rise in concern, research, and activism relating to the impacts of AI systems.”
The researchers note that significant work has gone into articulating ethical principles by many players involved with AI development, but the claims are meaningless without some way to verify them.
“People who get on airplanes don’t trust an airline manufacturer because of its PR campaigns about the importance of safety – they trust it because of the accompanying infrastructure of technologies, norms, laws, and institutions for ensuring airline safety.”
Among the core ideas put forward is to pay developers for discovering bias in algorithms. Such a practice is already widespread in cybersecurity; with many companies offering up bounties to find bugs in their software.
“Bias and safety bounties would extend the bug bounty concept to AI and could complement existing efforts to better document data sets and models for their performance limitations and other properties,” the authors wrote.
“We focus here on bounties for discovering bias and safety issues in AI systems as a starting point for analysis and experimentation but note that bounties for other properties (such as security, privacy protection, or interpretability) could also be explored.”
Another potential avenue is so-called “red teaming,” the creation of a dedicated team which adopts the mindset of a possible attacker to find flaws and vulnerabilities in a plan, organisation, or technical system.
“Knowledge that a lab has a red team can potentially improve the trustworthiness of an organization with respect to their safety and security claims.”
A red team alone is unlikely to give too much confidence; but combined with other measures can go a long way. Verification from parties outside an organisation itself will be key to instil trust in that company’s AI developments.
“Third party auditing is a form of auditing conducted by an external and independent auditor, rather than the organization being audited, and can help address concerns about the incentives for accuracy in self-reporting.”
“Provided that they have sufficient information about the activities of an AI system, independent auditors with strong reputational and professional incentives for truthfulness can help verify claims about AI development.”
The researchers highlight that a current roadblock with third-party auditing is that there’s yet to be any techniques or best practices established specifically for AI. Frameworks, such as Claims-Arguments-Evidence (CAE) and Goal Structuring Notation (GSN), may provide a starting place as they’re already widely-used for safety-critical auditing.
Audit trails, covering all steps of the AI development process, are also recommended to become the norm. The researchers again point to commercial aircraft, as a safety-critical system, and their use of flight data recorders to capture multiple types of data every second and provide a full log.
“Standards setting bodies should work with academia and industry to develop audit trail requirements for safety-critical applications of AI systems.”
The final suggestion for software-oriented methods of verifying AI ethics claims is the use of privacy-preserving machine learning (PPML).
Privacy-preserving machine learning aims to protect the privacy of data or models used in machine learning, at training or evaluation time, and during deployment.
Three established types of PPML are covered in the paper: Federated learning, differential privacy, and encrypted computation.
“Where possible, AI developers should contribute to, use, and otherwise support the work of open-source communities working on PPML, such as OpenMined, Microsoft SEAL, tf-encrypted, tf-federated, and nGraph-HE.”
The researchers, representing some of the most renowned institutions in the world, have come up with a comprehensive package of ways any organisation involved with AI development can provide assurance to governance and the wider public to ensure the industry can reach its full potential responsibly.
You can find the full preprint paper on arXiv here (PDF)
(Photo by Alexander Sinn on Unsplash)
“Leading AI researchers propose ‘toolbox’ for verifying ethics claims”