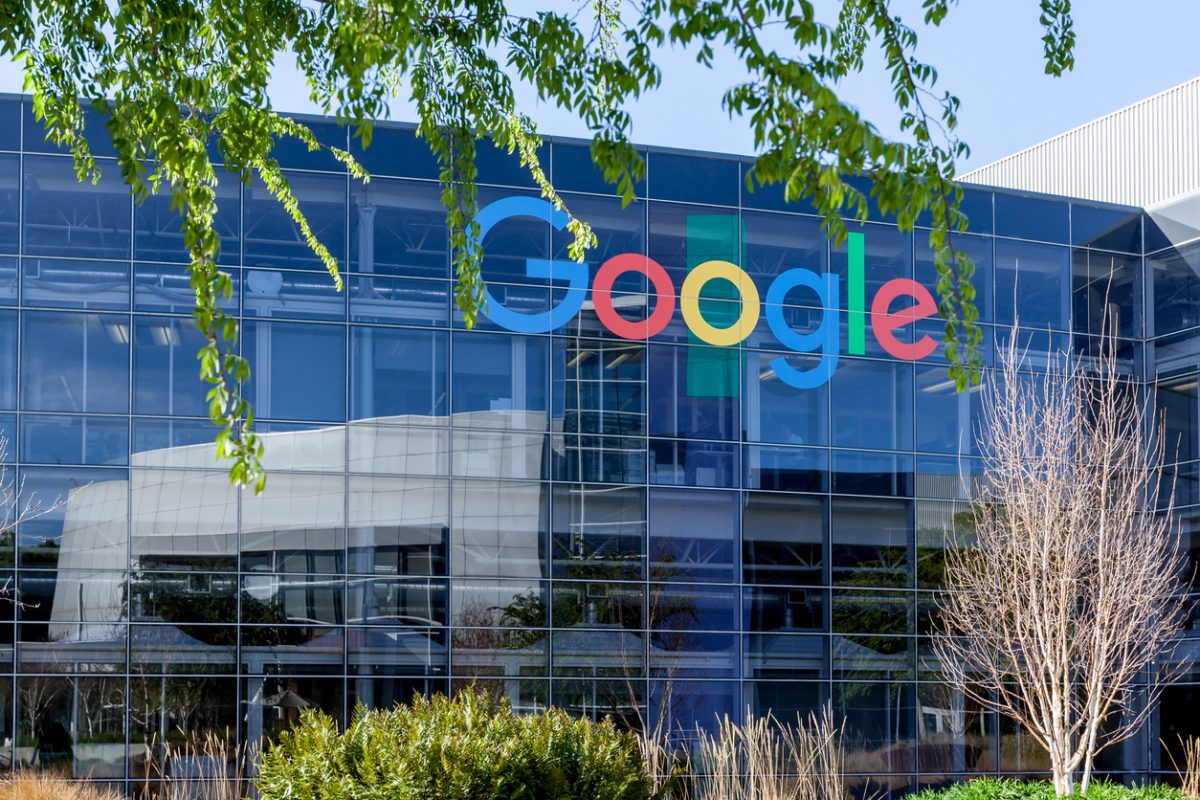
A team of AI researchers at Google has recently published a paper titled “Off-Policy Evaluation via Off-Policy Classification” on its blog. The paper talks about “off-policy classification” or OPC — as the researchers call it — which assesses the performance of AI-driven agents by treating evaluation as a classification problem.
The team says that their approach, which involves a variant of reinforcement learning that uses rewards to drive software policies toward goals, works with image inputs and scales to tasks, including vision-based robotic grasping.
Alex Irpan, software engineer at Google, said: “Fully off-policy reinforcement learning is a variant in which an agent learns entirely from older data, which is appealing because it enables model iteration without requiring a physical robot. With fully off-policy RL, one can train several models on the same fixed dataset collected by previous agents, then select the best one.”
In the blog, Google writes, OPC depends on two assumptions. The first is the final task has deterministic dynamics, which does not involve randomness in how states change, and the second is that the agent either succeeds or fails at the end of every trial. The paper proves that the performance of an agent is measured by how frequently its chosen action is an effective action, depending on how well the Q-function correctly classifies actions as effective versus catastrophic.
At its 2019 I/O Keynote last month, Google had announced that it has managed to condense 100GB of AI to just 0.5GB for a drastically sped-up Assistant. According to Scott Huffman, vice president of engineering at Google, the so-called “next generation” Assistant is so fast that it operates in real-time.

Attend the co-located AI & Big Data Expo events with upcoming shows in Silicon Valley, London, and Amsterdam to learn more. Co-located with the IoT Tech Expo, , and Cyber Security & .