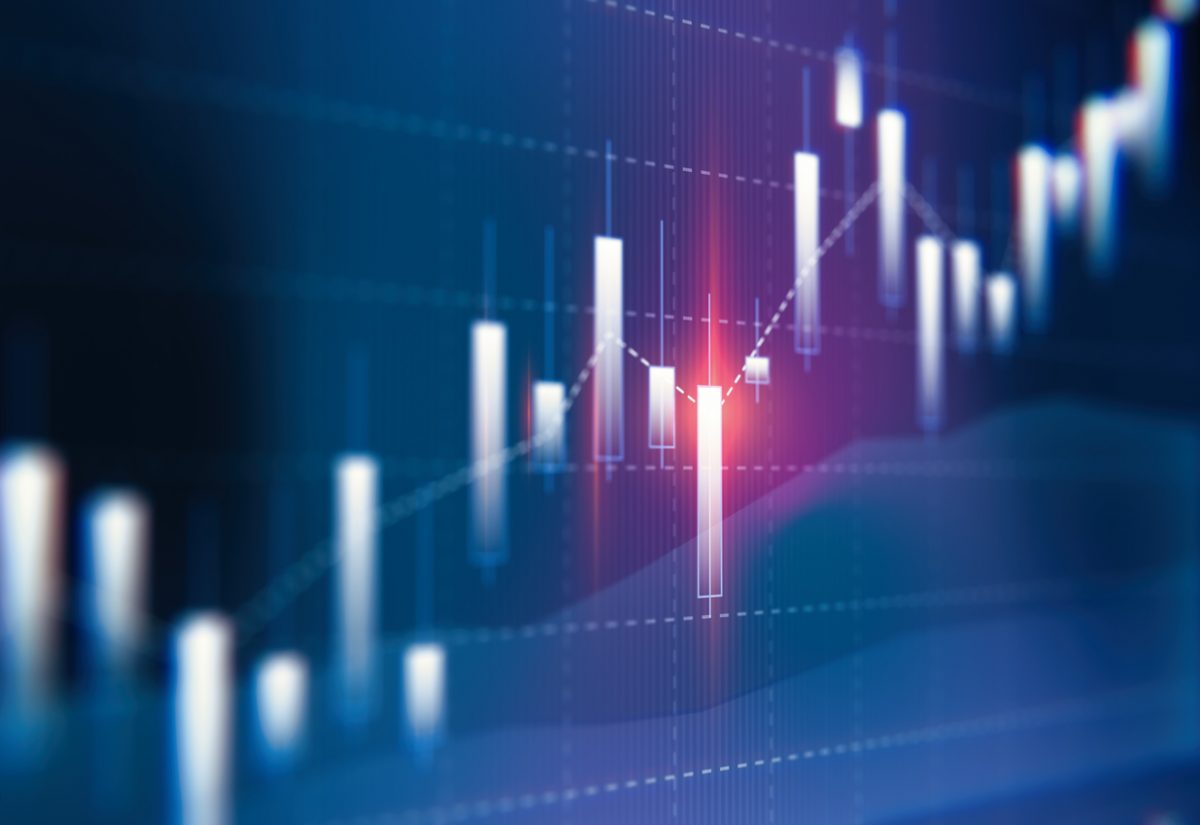
The promise of artificial intelligence (AI) and machine learning (ML) in an enterprise context is a tantalising one. In some ways, the combination of artificial intelligence and big data is helping transform legacy business intelligence systems – and potentially the executives who ran them.
But what best practices can be transferred between the two, and what happens from here? Kimberly Nevala (left) is director of business strategies at analytics, business intelligence and data management provider SAS. AI News sat down with Nevala prior to her appearance at the AI & Big Data Expo in San Francisco later this month to get the lowdown on what SAS is doing and will be doing in this space, and the marriage between big data and AI.
—
AI News: Could you define what your job role at SAS details and your day to day routine?
Kimberly Nevala: As a strategic advisor at SAS, my role encompasses market and industry research, content development, and advising our customers and prospects. In a nutshell, I help organisations understand the business potential and practical realities of emerging technologies such as AI and ML.
What are some of the initiatives you are working on at SAS with regards to AI and machine learning – and how are they impacting on customers?
We are very focused on not only the continuing evolution of AI capabilities but how AI/ML intersects with other domains to solve problems. This includes improving the IoT’s IQ through analytics. Some of our more recent work has included:
Making the IoT smart by marrying sensors and devices with streaming analytics. For example, Volvo and Mack Trucks are using sensor data from their on-road fleet to provide proactive real-time diagnostics 75% faster, resulting in less downtime and fewer critical breakdowns. The systems allow for rapid diagnostics and interventions from directing a driver to a local repair shop to uploading software updates while the truck is on the road. Similarly, Konica Minolta Japan sped up its PDCA (plan-do-check-act) cycle by deploying multiple analytical models incorporating sensor and traditional data sources to improve areas as diverse as malfunction forecasts and management optimisation.
The modern CAO needs to be fluent in decision science, not just data science
In the more traditional customer engagement space, Daiwa Securities was able to deliver a 2.7x increase in customer purchase rates and a 50% reduction in customer departure rates with their new CRM system by incorporating AI/ML.
We are also passionate about the use of Data (and AI) for Good. A great example is our work with WildTracks to create the Footprint Identification Tool (FIT). FIT enables the non-invasive monitoring of endangered animals like Cheetahs through digital analysis of their footprints.
How has the technology landscape altered over the past 10 years in your opinion in terms of the rise of artificial intelligence and big data – and the increasing combination of the two?
The intersection of AI and big data provides the ability to deliver more targeted, timely, relevant insight in a pervasive and intuitive manner (“Alexa, …”). Delivering that simplicity requires an analytics and data ecosystem that is markedly more complicated than 10 years ago.
To that end, effectively deploying analytics from BI to AI is now an exercise in portfolio management. Complete with discrete customer segments (executives to data scientists), diverse data environments (warehouses to lakes), development methods (deterministic report development to dynamic probabilistic algorithmic modeling) and a wide spectrum of deployment options (supercharged dashboards to streaming event detection and response).
How does this all relate to more traditional business intelligence therefore?
To be clear: traditional BI isn’t gone – it has just gotten smarter and easier to access. It is also a requisite part of a robust analytics portfolio. In fact, good discipline around BI (meaningfully measuring outcomes) is a key success factor for ensuring advanced AI/ML solutions deliver their intended value.
One of the eBooks you have written for SAS for ‘Portrait of a CAO’ back in 2014. What has changed between then and now? What difficulties would organisations looking to hire a chief analytics officer be facing today?
Analytics is increasingly integral to our business processes, products and services. Therefore, every executive today needs to be part CAO. That said, the complexity in the formal CAO role comes from the need to manage both technological and organisational change. The modern CAO needs to be fluent in decision science, not just data science. They also need to be collaborative – able to dynamically bring together collectives of internal and external experts to solve diverse problems. All while spearheading an elite analytics vanguard: continuously researching emerging solutions and enabling the organisation to decide if, when and how to realise value.
What is the most exciting use case you have seen focused around AI or ML?
The environment is so dynamic right now, it’s hard to choose. I’m most inspired by the work we are doing in the health care and public service domains. For example, projects like Healthy Nevada Project which aims to not just treat disease but promote health. This project is analysing genetic, clinical, environmental and socioeconomic data to better understand the complex interplay between these factors and health. There is also great work happening around rethinking how we serve and protect vulnerable populations.
Other use cases I find intriguing are from companies like Phylagen. Phylagen uses AI to analyse the microorganisms present on all things to identify where goods, materials and even people originated. This is a very different way of looking at the problem of supply chain integrity and even illicit trafficking. One that (from my perspective) doesn’t necessarily require the participation or the permission of the makers.
Effectively deploying analytics from BI to AI is now an exercise in portfolio management
I find the ability for AI/ML to enable a completely new approach to addressing a very old, complex problem striking.
What can we expect from SAS in the coming 12-18 months in this space?
Over the next 12-18 months we will continue to add additional analytics methods to the portfolio – with an emphasis on ML, DL, NLI and edge analytics. This includes features that enhance model interpretability and transparency. Also on the horizon: increasing usability and collaboration for all user types, continued enhancement of the integrated environment which supports the lifecycle from model development to deployment at scale (whether you code in SAS, Python, R, etc.) and embedding AI (including natural language interfaces) into our own solutions.
Finally, without giving too much away – what will the theme of your discussion be at AI & Big Data Expo later this month?
There are incredible synergies between the capabilities AI provides and the aspirations of digital transformation. AI also magnifies the challenges facing companies looking to “become digital”. This discussion will arm participants with the knowledge to successfully navigate these intersections.
Kimberly Nevala is speaking at the AI & Big Data Expo North America on November 28-29 at the Santa Clara Convention Center. Find out more about registering for the event by visiting here.
deepgeniusai.com