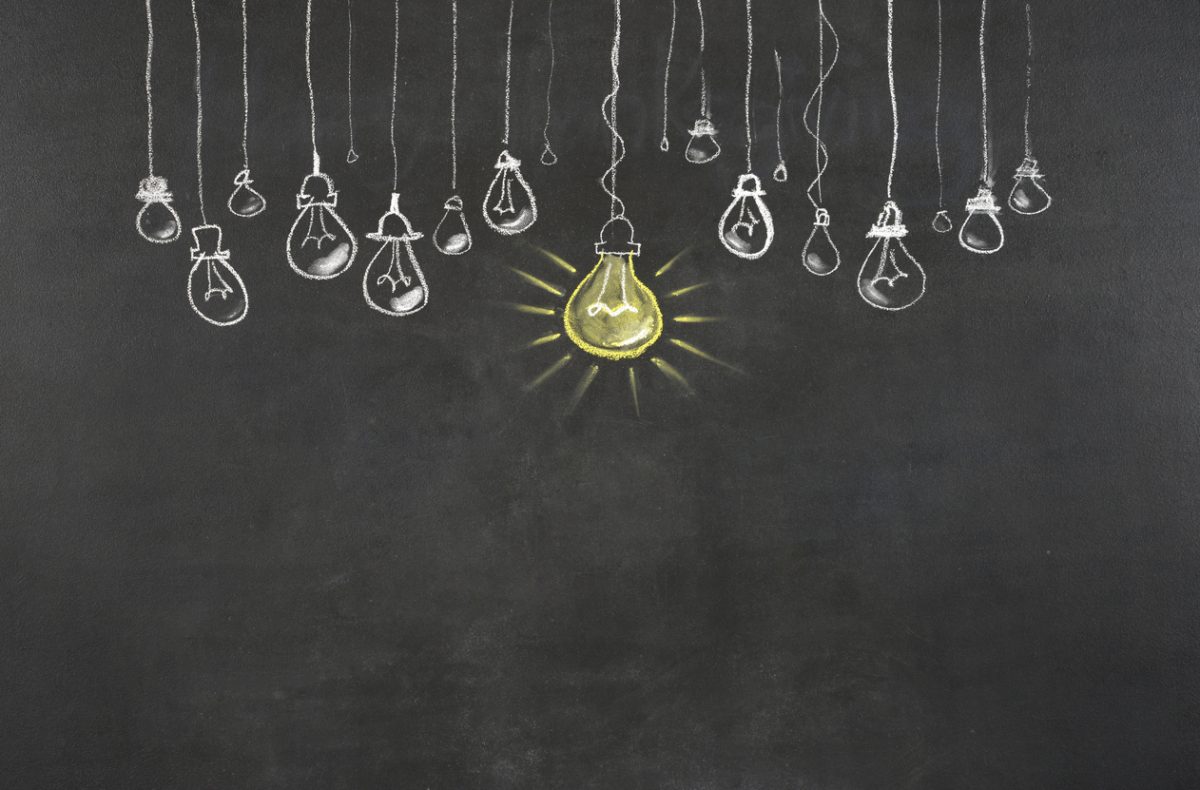
Academia has, and continues to play a fundamental role in artificial intelligence (AI) and machine learning (ML) development. As a Professor of Computer Science at the University of San Francisco as well as Chief Scientist at data integration software provider SnapLogic, I’ve been lucky to have worked with AI and ML in both an academic and professional capacity. I started my career as a pure academic, and only when I switched to my dual role some 10 years ago did I come to appreciate that, when it comes to driving innovation, industry and academia must to work together to realise significant benefits, especially with any technology that operates on data at scale.
Naturally, throughout the development of AI, academia is where a lot of the theoretical and experimental work has been accomplished. The challenge for academia has been access to real world usage data that can be used to train models as well as access to a platform in which AI and ML algorithms can be deployed and tested at scale.
About 15 years ago, search and social companies, finding existing database solutions unsuitable for their massive datasets, created custom solutions. With scalable storage systems in place, they then invited academics to analyse this newly collected data. These new types of vast data has helped power all of the theoretical machinery that had been developed in academia, and realise real recommendations and predictive applications. This increased availability of data, influenced the theoretical aspects of AI and created this symbiotic relationship between academia and industry. Without it, AI advances would likely not be as far along as they are today.
Evolving the relationship between industry and academia
In my role as both a professor and chief scientist for a technology company I have seen the culture differences between academia and industry first hand. In my view, the essence of both cultures have their place. Academia is a place for students to learn and discover the fundamentals of computer science and industry is a place to put the fundamentals into practice which results in real value to society. However, there are opportunities for both cultures to benefit from each other as collaboration increases to advance AI and ML.
I’ve been able to bring experiences from industry into my classes. Some of the things I teach now, especially in distributed systems, are things that you maybe wouldn’t traditionally see in academia. But, by teaching the realities of building production software, it better prepares students for a future in industry and it helps motivates them when learning difficult concepts. Having the link with industry also means some of my students have the opportunity to work on-site at SnapLogic, on real-world AI projects.
Similarly, the academic scientific method used in research is needed in the application of machine learning to real industry problems. Implementing machine learning is not like implementing new software functionality. For most functionality you can specify requirements and come up with a development schedule. This is not the case when trying to implement machine learning. Instead, machine learning requires lots of experimentation, which looks more a like an academic science experiment. In this way, the academic culture of establishing a hypothesis then running experiments is what is is needed to successfully deploy machine learning in industry.
AI in the business world
The benefits to business from AI will be far reaching in the next several years. Innovation, efficiency boosts and greater output will be chief amongst them. AI will help to free up valuable resources to be deployed in other areas, enabling the enhancement of existing products and services or the development of new ones. It will change job roles and allow workers to focus on tasks that rely on human skills, such as emotional insight or creative intelligence.
But this isn’t going to happen overnight. It’ll be a steady, incremental process. To keep us on course, collaboration between academia and industry will be essential. Together they’re stronger than the sum of their parts, and by sharing resources and talents, innovation in AI will thrive.